Unlocking Autonomy: What is Agentic AI and Why It Matters
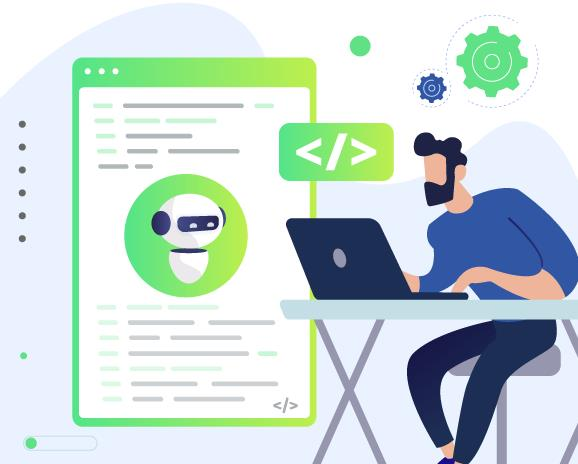
Imagine a world where artificial intelligence goes beyond simply responding to your commands. Picture AI that proactively identifies problems, sets its own goals, and takes independent action to solve them. This isn’t a scene from a futuristic movie; it’s the rapidly evolving reality of Agentic AI.
We’ve witnessed the transformative power of traditional AI and the creative prowess of generative AI. Now, a new wave of intelligence is emerging, one that imbues AI systems with a greater degree of autonomy and decision-making capability. This is Agentic AI, the next significant leap in artificial intelligence, poised to redefine how we interact with technology and conduct business across countless industries. In fact, Gartner projects that by 2028, a remarkable 33% of enterprise software applications will incorporate agentic AI, a staggering increase from less than 1% in 2024.
But the impact goes beyond mere integration. Gartner also forecasts that by 2028, agentic AI will autonomously make at least 15% of day-to-day work decisions, signifying a fundamental shift in how work gets done. Furthermore, the economic implications are substantial, with Gartner predicting a 25% reduction in customer service costs due to AI-driven automation.
So, what is Agentic AI exactly? It represents a paradigm shift from reactive and generative models to intelligent systems that can perceive their environment, reason about complex tasks, make independent decisions, and execute those decisions with minimal human oversight. Think of it as moving beyond tools that assist us to partners that can act on our behalf.
This blog post will serve as your comprehensive guide to understanding this exciting field. We will delve into the core definition of Agentic AI, explore its key characteristics that set it apart, differentiate it from related concepts like AI agents and generative AI, and illuminate its diverse real-world applications – including its exciting potential in software testing.
Defining Agentic AI: Beyond Traditional Paradigms
At its core, Agentic AI refers to artificial intelligence systems that can autonomously pursue specific goals by perceiving their environment, reasoning about actions, making decisions, and executing them with minimal human intervention. It’s about empowering AI to move beyond being a passive tool and to become an active agent.
To fully grasp the concept of Agentic AI, it’s crucial to understand its key characteristics:
- Autonomy: This is the defining feature. Agentic AI systems possess the capacity to initiate and complete tasks independently, without constant human oversight. They can self-direct and manage their own operations.
- Example: An Agentic AI system managing a smart home can adjust temperature and lighting based on occupancy and time of day, learning user preferences and adapting to changing conditions without explicit commands.
- Goal Orientation: Unlike traditional AI, which often focuses on specific tasks, Agentic AI is driven by objectives. It has the ability to define and work towards specific goals, breaking down complex tasks into manageable steps.
- Example: An Agentic AI designed for supply chain management can aim to optimize delivery times and costs. It can autonomously reroute shipments, negotiate with suppliers, and make decisions to achieve its objective.
- Reasoning: Agentic AI isn’t just about following pre-programmed rules. It has the capability to analyze situations, evaluate options, and make informed decisions based on context and available information. This allows it to handle unexpected situations and adapt to dynamic environments.
- Example: An Agentic AI in a customer service role can understand the nuances of a customer’s issue and determine the most appropriate solution, even if it wasn’t explicitly programmed for that specific scenario.
- Adaptability: The ability to learn from interactions and feedback is crucial for Agentic AI. These systems can adjust their strategies and actions based on experience, improving their performance over time.
- Example: An Agentic AI used for trading can analyze market trends in real-time and modify its investment strategies based on the outcomes of previous trades, constantly refining its approach.
- Interaction: Agentic AI is not meant to operate in isolation. It has the capacity to communicate and collaborate with humans and other AI agents to achieve goals. This collaborative aspect is essential for complex tasks that require diverse skills and knowledge.
- Example: An Agentic AI assistant can understand natural language queries and work with other specialized AI agents (e.g., an agent specializing in data analysis or another in content generation) to gather information and complete complex requests.
Agentic AI vs. Related Concepts: Clearing the Confusion
The landscape of artificial intelligence is rapidly evolving, and terms like Agentic AI, generative AI, and AI agents are often used, sometimes interchangeably. To truly understand what is Agentic AI, it’s crucial to distinguish it from these related but distinct concepts.
- Agentic AI vs. Generative AI: While both represent significant advancements in AI, their core functionalities and goals differ significantly.
- Generative AI focuses on creating new content – whether it’s text, images, code, audio, or video – based on patterns learned from vast datasets. Think of models like large language models (LLMs) that can write articles, create poems, or generate code snippets based on prompts. However, generative AI primarily responds to input and often requires human feedback for refinement and direction.
- Agentic AI, on the other hand, focuses on acting and making autonomous decisions to achieve specific goals. While an Agentic AI system might leverage generative AI as a tool to create content as part of its task (e.g., generating a report or drafting an email), its primary function is to orchestrate actions, reason through problems, and execute solutions independently.
- Analogy: Think of generative AI as a highly skilled artist who can paint a masterpiece based on your detailed instructions. Agentic AI is more like a project manager who not only understands the overall objective but can also plan the entire project, delegate tasks (potentially using generative AI for specific content creation), make strategic decisions along the way, and execute the plan autonomously.
- Agentic AI vs. AI Agents: The term “AI agent” is closely related to Agentic AI, but it’s important to understand the nuance.
- An AI agent is essentially a software entity that can perceive its environment through sensors, process that information, and act upon its environment through effectors to achieve specific goals. AI agents can range from simple rule-based systems to highly sophisticated, learning-based entities.
- Agentic AI is the underlying intelligence and architectural framework that empowers AI agents to exhibit a high degree of autonomy, reasoning, adaptability, and goal-directed behavior. In essence, Agentic AI describes the capabilities that make an AI agent truly autonomous and intelligent in its actions.
- Analogy: You can think of AI agents as individual workers with specific skills. Agentic AI is the management philosophy, the advanced cognitive abilities, and the underlying infrastructure that allows these workers to operate with greater independence, make strategic decisions, and coordinate effectively to achieve complex objectives. Not all AI agents are necessarily examples of sophisticated Agentic AI, but systems embodying Agentic AI are always comprised of one or more intelligent AI agents.
- Agentic AI vs. Traditional AI/Machine Learning: Traditional AI and machine learning models often require significant human intervention for complex tasks, adaptation to new environments, and decision-making beyond their initial training.
- Traditional AI might rely on pre-programmed rules and struggle with novel situations. Machine learning models excel at pattern recognition and prediction but typically require humans to define the tasks, prepare the data, and interpret the results.
- Agentic AI represents a step towards greater independence. While it still relies on machine learning and other AI techniques, it integrates them in a way that allows the system to learn and adapt autonomously, define its own sub-goals, and take actions without explicit human programming for every step. It’s about moving from systems that react to data to systems that act intelligently within their environment.
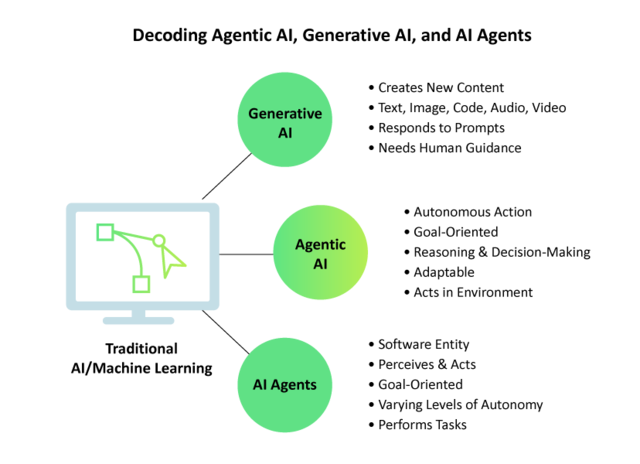
The Power of Autonomy: Real-World Applications of Agentic AI
The ability of Agentic AI systems to operate autonomously, reason effectively, and adapt to dynamic environments opens up a vast array of possibilities across numerous industries. Here are some key areas where Agentic AI is already showing promise and is poised for significant growth:
- Customer Service: Imagine AI agents that go beyond simple chatbots. Agentic AI can power customer service representatives that truly understand complex customer issues, proactively access and synthesize information from various sources, and autonomously resolve problems from start to finish, leading to faster, more personalized, and more effective support.
- Example: An Agentic AI customer service agent can handle an insurance claim by understanding the customer’s situation, accessing policy details, gathering necessary documentation, communicating with relevant departments, and ultimately processing the claim – all with minimal human intervention.
- Healthcare: Agentic AI can revolutionize healthcare by creating intelligent systems that can monitor patients remotely, analyze complex medical data to identify patterns and predict potential health issues, suggest personalized treatment plans based on a holistic understanding of a patient’s condition, and even optimize resource allocation within hospitals for greater efficiency.
- Example: An Agentic AI system could continuously monitor a patient’s vital signs through wearable devices, detect subtle anomalies that might indicate an impending health crisis, and proactively alert medical professionals, potentially saving lives.
- Finance: The financial industry can leverage Agentic AI for a multitude of tasks, including automated high-frequency trading that reacts to market fluctuations in real-time, sophisticated fraud detection systems that learn and adapt to evolving threats, personalized financial advisors that understand individual goals and provide tailored recommendations, and more efficient risk management strategies.
- Example: An Agentic AI trading agent can analyze vast amounts of market data and execute trades based on complex algorithms and learned patterns, often outperforming human traders in speed and efficiency.
- Supply Chain Management: Optimizing complex supply chains requires constant monitoring, prediction, and adaptation. Agentic AI can create autonomous systems that can predict demand fluctuations, manage inventory levels dynamically, optimize logistics and routing for efficiency, and proactively respond to disruptions like weather events or supplier issues, ensuring smoother and more resilient supply chains.
- Example: An Agentic AI managing a logistics network can autonomously reroute deliveries in response to a traffic jam or a sudden warehouse closure, minimizing delays and costs.
- IT Operations: Managing modern IT infrastructure is increasingly complex. Agentic AI can power autonomous IT operations agents that can proactively identify and resolve system issues before they cause downtime, automate routine maintenance tasks, enhance cybersecurity by detecting and responding to threats in real-time, and optimize resource allocation for peak performance.
- Example: An Agentic AI monitoring a server network can detect unusual activity that might indicate a security breach and automatically implement countermeasures to neutralize the threat.
- Smart Homes and Buildings: Agentic AI is the key to truly intelligent environments. Autonomous agents can learn user preferences and automatically adjust lighting, temperature, security systems, and entertainment based on routines, occupancy, and even mood, creating more comfortable, efficient, and personalized living and working spaces.
- Example: An Agentic AI in a smart home can learn your preferred temperature at different times of the day and automatically adjust the thermostat accordingly, while also anticipating your arrival and turning on lights and music.
- Research and Development: Accelerating scientific discovery requires sifting through vast amounts of data and generating new hypotheses. Agentic AI can act as a powerful research assistant, autonomously analyzing scientific literature, identifying patterns and connections, suggesting new research directions, and even helping to design and execute experiments.
- Example: An Agentic AI in a pharmaceutical research lab can analyze millions of research papers to identify potential drug candidates for a specific disease, significantly speeding up the discovery process.
- Software Testing: For companies focused on software quality, Agentic AI offers a transformative potential by enabling the creation of autonomous testing agents. These agents can:
- Intelligently Design Test Cases: Analyze requirements, user stories, and code to automatically generate a comprehensive suite of test cases, including edge cases and scenarios humans might miss.
- Example: An Agentic AI testing agent can understand the functionality of a new user authentication feature and autonomously create test cases covering valid and invalid credentials, password reset flows, and account lockout scenarios.
- Self-Healing Test Scripts: Detect changes in the application UI or functionality and automatically update test scripts, significantly reducing the time and effort spent on test maintenance.
- Example: If the label of a button changes from “Submit” to “Save,” an Agentic AI testing agent can automatically identify this change and update the corresponding test script without manual intervention.
- Autonomous Test Execution and Analysis: Execute test suites across various environments and automatically analyze the results, identifying bugs, pinpointing the root cause of failures, and providing insightful reports for developers.
- Example: An Agentic AI can run a full regression test suite after a code deployment and autonomously analyze the logs and identify newly introduced bugs, providing developers with detailed information for debugging.
- Exploratory Testing: Simulate user behavior and explore the application in an intelligent and unpredictable manner to uncover usability issues and unexpected bugs that predefined test cases might miss.
- Example: An Agentic AI agent can be tasked with “exploring the checkout process” of an e-commerce site and will autonomously navigate through different options, try various input combinations, and identify potential issues in the user experience.
- Performance and Load Testing Optimization: Dynamically adjust testing parameters based on real-time system performance metrics to identify bottlenecks and scalability issues more effectively than traditional static load tests.
- Example: An Agentic AI conducting load testing can automatically increase the number of virtual users until response times exceed a certain threshold, precisely identifying the system’s breaking point.
- Security Vulnerability Detection: Proactively identify potential security flaws by autonomously probing the application with various simulated attack vectors and analyzing the responses for vulnerabilities.
- Example: An Agentic AI security testing agent can automatically try common cross-site scripting (XSS) or SQL injection attacks on different input fields to identify potential security weaknesses.
- Intelligently Design Test Cases: Analyze requirements, user stories, and code to automatically generate a comprehensive suite of test cases, including edge cases and scenarios humans might miss.
Navigating the Challenges and Embracing the Future of Agentic AI
While the potential of Agentic AI is immense, its development and widespread adoption are not without their challenges. Addressing these hurdles is crucial to ensuring the responsible and beneficial integration of autonomous intelligence into our lives and businesses.
Current Challenges and Limitations of Agentic AI
- Reliability and Predictability: Ensuring that Agentic AI systems behave consistently and predictably, especially in complex and safety-critical situations, is paramount. We need to build trust in their decision-making processes.
- Transparency and Explainability: Understanding why an Agentic AI agent made a particular decision (often referred to as the “black box problem”) is critical for accountability, debugging, and building user trust. Developing methods for explainable AI (XAI) is crucial.
- Security and Privacy: As Agentic AI systems become more integrated into our lives and handle sensitive data, ensuring their security against malicious attacks and safeguarding user privacy are of utmost importance.
- Ethical Considerations: The increasing autonomy of Agentic AI raises significant ethical questions. We need to address potential biases embedded in data and algorithms, ensure fairness in decision-making, and clearly define responsibility and accountability for the actions of autonomous agents.
- Infrastructure and Scalability: Developing and deploying sophisticated Agentic AI systems often requires significant computational resources, advanced infrastructure, and robust data management capabilities. Scalability to handle widespread adoption is also a key consideration.
- Governance and Regulation: As Agentic AI becomes more powerful, establishing appropriate governance frameworks and regulations will be necessary to guide its development and deployment in a way that aligns with societal values and mitigates potential risks.
The Future Trends and Potential Advancements in Agentic AI
Despite these challenges, the future of Agentic AI is incredibly promising. We can anticipate several key trends and advancements:
- Enhanced Autonomy and Reasoning: Future Agentic AI systems will exhibit even greater levels of autonomy, capable of tackling more complex tasks with more sophisticated reasoning and problem-solving abilities. They will be able to learn and adapt in increasingly nuanced and dynamic environments.
- Improved Collaboration: We will see advancements in how humans and Agentic AI agents collaborate seamlessly. Natural language interfaces and intuitive interaction methods will become more sophisticated, fostering true partnership. Furthermore, different AI agents will become better at coordinating and working together to achieve common goals.
- Personalization and Contextual Awareness: Future Agentic AI will have a deeper understanding of individual needs, preferences, and contexts. They will be able to personalize experiences and provide highly tailored solutions based on a rich understanding of the user and their environment.
- Integration with Emerging Technologies: The power of Agentic AI will be amplified by its integration with other cutting-edge technologies such as advanced robotics, the Internet of Things (IoT), and quantum computing, leading to entirely new applications and capabilities.
- New Applications and Industries: As the field matures, we can expect to see Agentic AI emerge in currently unimagined applications and transform industries we haven’t even considered yet. Its ability to automate complex cognitive tasks will unlock new levels of efficiency and innovation.
Remember! Humans are Still Import
It’s crucial to remember that the development and deployment of Agentic AI should be guided by a human-centric approach. The focus should be on how these intelligent systems can augment human capabilities, solve real-world problems effectively, and ultimately benefit society as a whole, while carefully considering and mitigating potential risks.
Based on the document, here’s a section that introduces Qyrus AI Agents for the blog:
Qyrus AI-Verse: A Suite of Intelligent Agents for Testing
Qyrus leverages the power of AI through its Qyrus AI-Verse, a collection of Single Use Agents (SUAs) designed to address specific challenges within the software quality assurance process. These AI-driven agents empower testers and innovators to achieve better outcomes throughout the Software Development Life Cycle (SDLC) by utilizing autonomous algorithms to test, self-heal, and predict tests.
Here’s a brief overview of some of the key Qyrus AI Agents:
- Qyrus TestPilot: Acts as an AI co-pilot, enabling users to create and execute real-time tests directly from any URL and automatically generates relevant test cases.
- TestGenerator & TestGenerator+: Automatically transforms JIRA tickets into actionable test scenarios, with TestGenerator+ expanding coverage to explore untested areas and proactively identify gaps.
- API Builder: Instantly virtualizes APIs, converting user requirements into testable endpoints to facilitate parallel development and testing.
- Echo: Generates synthetic, realistic data to fulfill diverse testing requirements.
- Rover: Functions as an autonomous AI scout, exploring applications and identifying anomalies at a much faster pace than human testers.
- Qyrus Eval: Evaluates AI model outputs for consistency and appropriateness, proving particularly useful in conversational AI applications.
Qyrus also provides agents like Manual Executor, DomainLens, and TestBridge Converter & Import to support manual testing with AI suggestions, convert document-based knowledge into test scenarios, and translate coded tests to Qyrus keywords and vice versa. Additionally, agents like Insights & AnalytiQ, API Discovery, Healer, BotMetrics, Visual Testing, and UXtract offer capabilities for performance insights, automated test updates, chatbot evaluation, UI/UX consistency, and enhanced interaction testing.
These AI agents contribute to significant improvements, including a 70% faster test creation process, increased efficiency leading to 35% or more cost savings, and a 70% increase in collaboration.
Conclusion: The Autonomous Horizon
The emergence of Agentic AI signifies a profound shift in the evolution of artificial intelligence. It moves us beyond systems that merely process information or generate content towards intelligent agents capable of independent thought, action, and adaptation. This paradigm shift promises to unlock unprecedented levels of efficiency, innovation, and problem-solving capabilities across virtually every sector.
As Agentic AI continues to mature, it holds the key to automating complex cognitive tasks, augmenting human intellect, and driving advancements that were once considered the realm of science fiction. Embracing this autonomous horizon requires not only technological innovation but also careful consideration of ethical implications, security measures, and the development of robust governance frameworks.
Ready to Explore the Power of Agentic AI in Software Testing?
At Qyrus, we recognize the transformative potential of Agentic AI and are actively leveraging its capabilities to revolutionize the field of software testing. We believe that autonomous testing agents can significantly enhance efficiency, improve test coverage, and accelerate the delivery of high-quality software.
If you are intrigued by the possibilities of integrating Agentic AI into your software testing processes and want to explore how Qyrus is pioneering this technology, we invite you to contact us today for a discussion. Let us show you how autonomous intelligence can elevate your testing strategy and help you build better software, faster.